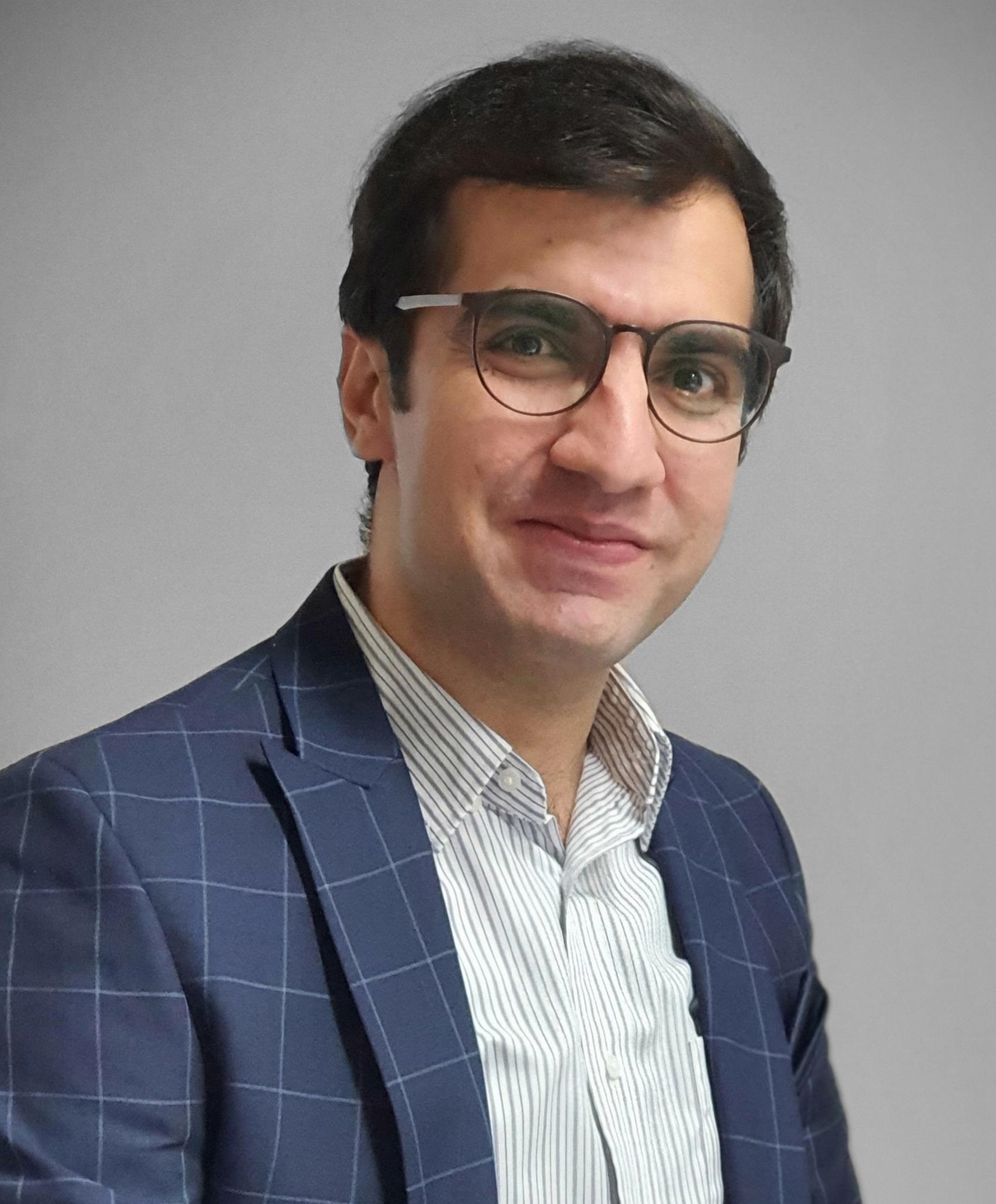
Ihsan Ullah Khan, PhD
Robotics Engineering
- Daegu
- DGIST
- ResearchGate
- Github
- YouTube
- Google Scholar
You May Also Enjoy
M.S Defense Glimps
less than 1 minute read
Published:
This is a sample blog post. Lorem ipsum I can’t remember the rest of lorem ipsum and don’t have an internet connection right now. Testing testing testing this blog post. Blog posts are cool.
Blog Post number 3
less than 1 minute read
Published:
This is a sample blog post. Lorem ipsum I can’t remember the rest of lorem ipsum and don’t have an internet connection right now. Testing testing testing this blog post. Blog posts are cool.
Blog Post number 2
less than 1 minute read
Published:
This is a sample blog post. Lorem ipsum I can’t remember the rest of lorem ipsum and don’t have an internet connection right now. Testing testing testing this blog post. Blog posts are cool.
ADVENT - Adversarial Entropy Minimization for Domain Adaptation in Semantic Segmentation
8 minute read
Published:
This post describes the recent work on unsupervised domain adaptation for semantic segmentation presented at CVPR 2019. ADVENT is a flexible technique for bridging the gap between two different domains through entropy minimization. Our work builds upon a simple observation: models trained only on source domain tend to produce over-confident, i.e., low-entropy, predictions on source-like images and under-confident, i.e., high-entropy, predictions on target-like ones. Consequently by minimizing the entropy on the target domain, we make the feature distributions from the two domains more similar. We show that our approach achieves competitive performances on standard semantic segmentation benchmarks and that it can be successfully extended to other tasks such as object detection.